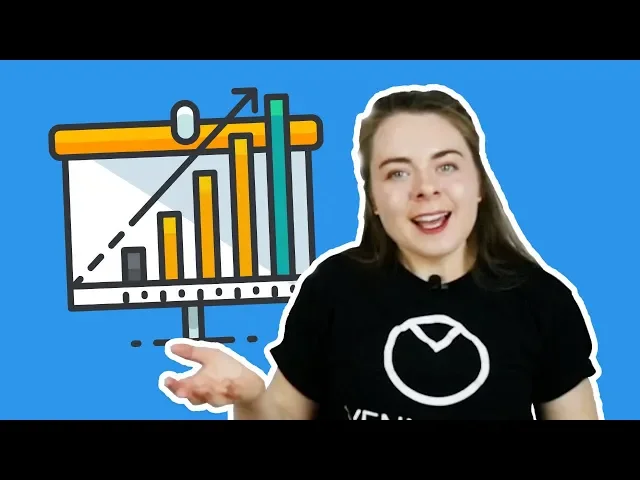
Types of graphs and when to use them
Business Intelligence
May 18, 2025
Choosing the right graph type is essential for effective data visualization. Explore common graph types and their best uses for clear insights.

When it comes to understanding data, choosing the right graph is critical. Here's a quick breakdown of common graph types and when to use them:
Bar Charts: Best for comparing categories. Example: Sales by region.
Line Graphs: Ideal for showing trends over time. Example: Monthly revenue growth.
Scatter Plots: Use to find relationships between two variables. Example: Ad spend vs. conversions.
Pie Charts: Great for visualizing parts of a whole. Example: Market share distribution.
Heatmaps: Perfect for spotting patterns in large datasets. Example: Website click intensity.
Quick Comparison Table
Graph Type | Best Use | Strength |
---|---|---|
Bar Charts | Comparing categories | Simple and easy to read |
Line Graphs | Showing trends over time | Clearly shows progression |
Scatter Plots | Identifying relationships | Highlights correlations |
Pie Charts | Showing proportions | Visualizes part-to-whole data |
Heatmaps | Displaying data intensity | Uncovers patterns effectively |
Key takeaway: The right graph can transform complex data into clear insights, making it easier to communicate trends, relationships, and patterns effectively.
Basic Graph Types and Their Uses
Bar Charts: Comparing Categories
Bar charts are fantastic for comparing different categories, thanks to their simple and intuitive design. The rectangular bars make it easy to see numerical differences at a glance, as our eyes naturally compare their lengths against a shared baseline [3].
"Bar charts enable us to compare numerical values like integers and percentages. They use the length of each bar to represent the value of each variable." - Tableau [5]
To create effective bar charts, keep these tips in mind:
Always start the baseline at zero for accurate comparisons.
Use consistent rectangular shapes for clarity.
Sort bars by length unless the categories follow a natural order.
Use color thoughtfully to highlight key insights.
For example, in website traffic analysis, bar charts can illustrate which channels - like search engines, social media, or email - generate the most visits [4].
Line Graphs: Tracking Time Trends
Line graphs are perfect for showing how data changes over time. They help uncover trends, patterns, and even seasonal shifts by connecting data points in a continuous flow [6]. Whether you're tracking sales over months or temperature changes across seasons, line graphs make trends easy to spot.
Here’s how to keep line graphs clear and effective:
Limit the number of lines to five or fewer to avoid clutter [7].
Use clear axis labels with appropriate units.
Add light gridlines to make values easier to read.
Represent missing data points with dotted lines to maintain transparency [8].
These graphs are indispensable for time-based data analysis, helping you identify gradual changes or sudden spikes with ease.
Scatter Plots: Finding Relationships
Scatter plots are a go-to tool for exploring relationships between two numeric variables. By plotting individual data points, they help uncover correlations, clusters, and outliers [9].
For instance, a telecommunications company analyzed the link between a self-service feature and customer retention using a scatter plot. They plotted feature adoption rates against retention rates and identified clusters showing that higher adoption aligned with lower churn [10]. Similarly, HR teams have used scatter plots to evaluate training investments, comparing costs to productivity scores to identify the most impactful programs [10].
These plots are invaluable when you need to dig into relationships between variables and make data-driven decisions.
Pie Charts: Showing Parts of a Whole
Pie charts are ideal for visualizing percentage distributions and proportional relationships. They’re best used when:
You have fewer than seven categories.
The values add up neatly to 100%.
There are clear differences between the values.
When used appropriately, pie charts provide a straightforward way to show how each part contributes to the whole.
Heatmaps: Displaying Data Intensity
Heatmaps use color intensity to represent data values, making them excellent for spotting patterns and concentrations in large datasets. They’re particularly effective for visualizing data like:
Website click patterns.
Sales performance across regions.
Customer engagement levels.
Time-based activity trends.
Heatmaps are a powerful way to identify critical areas of engagement or operational hotspots, helping businesses focus their attention where it matters most.
Specialized Graphs for Complex Data
Tree and Hierarchy Charts
Tree and hierarchy charts are perfect for visualizing nested relationships and organizational structures. They take broad categories and break them down into detailed levels, making them ideal for tasks like decision-making, project planning, and presenting complex ideas in a clear way.
"Tree diagrams are versatile and useful for decision-making and other tasks across various fields and industries, including marketing, software development, logistics, and project management" [12]
A real-world example? Back in 2001, the Pearl River, NY School District used a tree diagram to link their strategic objectives with measurable outcomes [11].
When creating tree charts, follow these principles to get the best results:
Clearly define your goal.
Break each level into logical components.
Make sure all elements are both necessary and sufficient.
Maintain consistent levels of hierarchy throughout.
Next, let’s move beyond hierarchies and dive into network maps, which uncover broader interdependencies.
Network and Connection Maps
Unlike tree charts, which focus on nested structures, network maps are all about showing connections and dependencies. They’re incredibly useful for understanding how systems interact and for pinpointing issues in interconnected setups.
"Network visualization is our industry's embodiment of the phrase 'A picture is worth a thousand words.'" - Steve Petryschuk [13]
For instance, imagine a food contamination case. Using tools like ArcGIS Knowledge, investigators can trace contaminated ingredients through supply chains. By connecting reports of foodborne illness to restaurants, menu items, and suppliers, they can identify the source of the problem [14].
Distribution and Statistical Graphs
Distribution and statistical graphs are your go-to tools for analyzing data patterns and relationships. Common types include histograms, box plots, and frequency polygons, each tailored for specific analytical needs.
Here’s a quick guide to when and why to use each:
Graph Type | Best Used For | Key Advantage |
---|---|---|
Histogram | Continuous data distribution | Highlights frequency patterns |
Box Plot | Identifying outliers | Compares distributions effectively |
Frequency Polygon | Comparing multiple datasets | Great for visualizing trends |
To give you an idea, in a normal distribution, about 68% of values fall within one standard deviation from the mean [16]. When working with these graphs, here are some tips to keep in mind:
Select histogram widths carefully to clearly show the shape of the distribution.
Use frequency polygons when comparing trends across multiple datasets.
Rely on box plots to spotlight outliers and differences in distributions.
That said, while box plots are excellent for identifying extreme values, they can sometimes miss finer details. Pairing them with histograms can give you a more complete view of the data [15].
Selecting the Best Graph Type
Graph Selection Guide
Choosing the right graph depends on the nature of your data and the message you want to convey. Here's a quick reference table to help you decide:
Goal | Best Graph Type | When to Use |
---|---|---|
Comparison | Bar Charts | To compare values across categories or time periods. |
Time Trends | Line Graphs | To illustrate continuous changes over time. |
Relationships | Scatter Plots | To analyze correlations between two variables. |
Composition | Pie Charts | To show parts of a whole (best for simple proportions). |
Distribution | Histograms | To explore data spread and patterns. |
"Data is only useful and valuable if you know how to properly visualize it and give it context." - Infogram [1]
When deciding, take into account factors like the size of your dataset, the types of variables, your audience's needs, and the key insights you aim to highlight.
Research by Cleveland and McGill offers insight into how visual elements influence our ability to interpret data. They identified a hierarchy of visual perception, ranked from easiest to most challenging [17]:
Position along a common scale
Position along non-aligned scales
Length
Angle and slope
Area
Volume, density, and color saturation
Color hue
Understanding this hierarchy can help you design visuals that are both clear and impactful. Up next, let's explore common design mistakes to avoid when creating graphs.
Graph Design Mistakes to Avoid
Even the best data can be misinterpreted if visualized poorly. Here are some common pitfalls to steer clear of:
Mismatched Graph Types: Pie charts are not ideal for comparisons. Use bar or line charts for clearer insights [19].
Visual Complexity: Overly complicated visuals can confuse your audience. Stick to clean, focused designs. If your data is complex, consider using multiple charts to break it down [18]. Research shows that simpler charts improve decision-making [20].
Scale Distortion: Misleading axis scales can distort your message. Always use zero-baselines and consistent measurements to maintain accuracy.
How to Pick the RIGHT Charts For Your Data [TYPES OF GRAPHS AND CHARTS]
AI-Powered Data Visualization
AI is reshaping how we interact with data, turning raw numbers into meaningful visuals through automation and natural language processing. By enhancing graph selection, streamlining updates, and simplifying user interactions, AI-powered tools make it easier to uncover actionable insights from complex datasets.
Smart Graph Recommendations
AI-driven visualization tools can analyze the structure of your data and suggest the best chart types to represent it. For instance, if you're reviewing departmental spending, AI can identify relationships between categories and numbers, assess distribution patterns, and recommend the most effective visualizations to highlight the data's story [22].
But AI doesn't stop at recommendations - it also plays a key role in managing data in real time.
Live Dashboard Updates
Keeping data accurate and up-to-date is critical for effective visualizations, and AI-powered dashboards excel in this area. With global data generation expected to surpass 180 zettabytes annually by 2025 [23], these dashboards handle the challenge by:
Automating data cleaning to ensure accuracy
Recognizing patterns to surface relevant trends
Offering predictive insights for forward-looking analysis
These features ensure your visualizations stay relevant and reliable, even as data volumes grow.
Simple Language Controls
One of the most user-friendly advancements in AI-powered visualization is the ability to translate plain language into technical queries. Users can simply say things like "compare with industry averages" or "show monthly breakdown", and the AI will adjust the visualization to meet the request [22].
This contextual understanding allows for complex data transformations with minimal effort, making data analysis accessible to everyone. Whether you're a seasoned analyst or a team member with no technical background, these tools empower you to explore data and uncover insights independently [22][24].
Key Points to Remember
Here’s a quick recap to help refine your data visualization approach.
Effective data visualization starts with selecting the right type of graph for your audience. Why? Because the human brain processes visuals 60,000 times faster than text [2].
To create impactful visualizations, focus on these three critical factors:
Data Structure: Understand whether your data is categorical or numerical, and evaluate its volume [17].
Audience Needs: Adapt your presentation to match your audience's level of understanding [17].
Visualization Goal: Define the specific action or insight you want your audience to take away [25].
"Numbers are meaningless without context. People need to compare a number against another number in order to interpret it."
Kate Moran, NN/g [25]
Best Practices for Clear Visuals
To ensure your visualizations guide effective decision-making, prioritize clarity:
Use color wisely: Highlight important details without overwhelming your audience [26].
Keep order consistent: When presenting multiple graphs, maintain a logical flow [26].
Include zero-baseline y-axes: This ensures your data isn’t misleading [21].
Avoid Common Pitfalls
Even the most polished visualizations can fall short if these mistakes creep in:
Skip 3D effects, which often obscure information.
Focus only on metrics that are essential for decision-making.
Use proper scales to ensure accuracy and avoid misinterpretation [19][21].
"Data visualization is the language of decision-making. Good charts effectively convey information. Great charts enable, inform, and improve decision-making."
Dante Vitagliano [21]
Why It Matters
Companies that effectively use business intelligence tools can act on insights without IT involvement 48% of the time [27]. The real power of visualization lies in its ability to deliver quick, precise insights that lead directly to action. By tailoring your visuals for clarity and purpose, you can transform data into a powerful decision-making tool.
FAQs
What type of graph should I use to show both time trends and category comparisons?
When you need to showcase time trends alongside category comparisons, a line chart is a go-to option. These charts excel at illustrating changes over time, and by using separate lines for each category, they make it straightforward to compare trends across different groups.
For a deeper perspective, consider a combination chart. In this setup, bars represent the categorical data, while a line overlays the time-based trend. This dual format emphasizes both category differences and overall trends, offering a clearer and more comprehensive view of your data.
What are common mistakes to avoid when creating clear and accurate data visualizations?
To design data visualizations that truly communicate insights, there are a few common traps you’ll want to sidestep. One major issue is overloading your charts with unnecessary complexity or clutter. When a chart is too busy, it can overwhelm your audience and bury the key takeaways. Aim for simplicity - highlight the data points that matter most.
Another frequent misstep is picking the wrong type of chart for your data. For instance, if you're looking to show trends over time, a pie chart isn’t going to cut it. Instead, choose a format that aligns with the story your data is trying to tell, like a line or bar graph for time-based comparisons.
Lastly, don’t forget to include crucial context in your visuals. Clear labels, baselines, and units of measurement are non-negotiable. Without these, your audience might struggle to grasp the true meaning behind the numbers. By steering clear of these pitfalls, you’ll craft visualizations that not only look great but also empower informed, data-driven decisions.
How can AI tools help in choosing and updating data visualizations?
AI tools make choosing and updating data visualizations much easier by analyzing your dataset and recommending the best type of chart or graph for your needs. For instance, they can determine whether a bar chart, line graph, or scatter plot is the most effective way to present your data. This not only saves time but also improves how clearly your data tells its story.
These tools also simplify updates by connecting to real-time data. As new information comes in, visualizations adjust automatically, keeping your dashboards accurate and current without any manual effort. This means you can make quicker, informed decisions based on up-to-date insights.