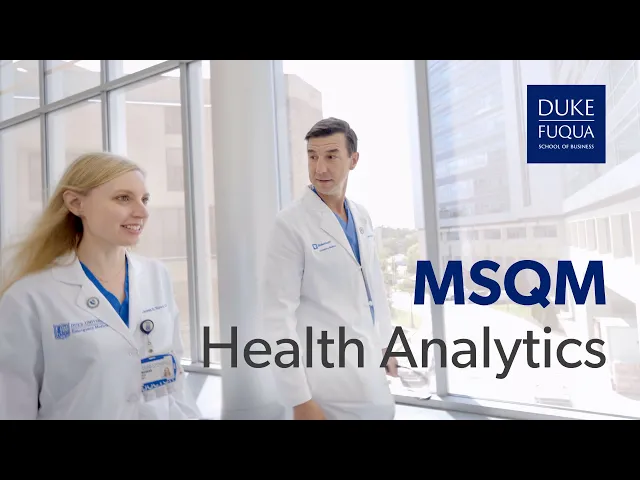
AI BI in Healthcare: Case Study Insights
Business Intelligence
Apr 23, 2025
Explore how AI-powered business intelligence is revolutionizing healthcare through faster insights, improved accuracy, and enhanced decision-making.

AI-powered business intelligence (BI) is transforming healthcare by delivering faster insights, improving accuracy, and empowering staff to analyze data independently. Here's what you need to know:
Faster Analysis: Reduces data processing time from weeks to minutes.
Key Benefits: Enhances billing accuracy, supports faster diagnoses, and optimizes staffing and inventory management.
Proven Results: Hospitals saved an average of $45,000 annually and improved productivity.
How It Works: AI-driven BI connects directly to hospital databases, enabling real-time data analysis through interactive dashboards, self-service tools, and collaborative workspaces.
Case Studies:
Billing Audits: Automated processes reduced errors and sped up claims resolution.
Diagnostics: AI-assisted imaging reviews improved decision-making.
Resource Planning: Predictive tools optimized staffing and supply management.
Focus on integrating data, fostering team collaboration, and providing targeted training to achieve similar results.
Business, AI, and Health Care: Inside Duke's Innovative ...

How AI-Driven BI Works in Healthcare
Building on the operational improvements mentioned earlier, let’s break down how these platforms work behind the scenes. Hospitals use AI-powered BI platforms that connect directly to their databases, enabling real-time analysis and support for decision-making.
Key Features of BI Platforms
These platforms use AI to interpret data, provide context, and create reports. Some standout features include:
AI-powered queries for instant insights
Context-aware reporting tailored to specific needs
Real-time KPI dashboards for tracking performance metrics
User Interfaces for Healthcare Teams
Healthcare professionals interact with these systems through:
Interactive dashboards showing bed occupancy and resource usage
Self-service query tools for customized data exploration
Shared workspaces to collaborate on data analysis
These features lay the groundwork for the success stories discussed in the next section.
3 Hospital Success Stories
Here are three examples that show how these solutions have made a real difference.
Case 1: Streamlining Administrative Tasks
A regional hospital in Florida implemented Querio to connect its electronic health records with an easy-to-use query system. By automating billing audits, the hospital quickly identified discrepancies, improving claims processing and accuracy.
Key results:
Better insight into billing processes
Less time spent manually reviewing claims
Faster billing resolutions, leading to happier patients
This example highlights how automation can speed up processes and reduce errors.
Case 2: Improving Diagnostic Efficiency
A teaching hospital in New England integrated Querio with radiology and clinical data systems. AI tools analyzed past imaging reports alongside current test results, helping flag issues and suggest potential diagnoses. This allowed radiologists to focus on more complex cases.
Key results:
Faster initial case reviews
Increased confidence in diagnostic decisions
Reduced need for unnecessary follow-up procedures
This approach saves time and supports more reliable diagnoses.
Case 3: Smarter Staffing and Inventory Management
A large health system used Querio to unify admissions and inventory data. Predictive analytics and dynamic dashboards helped administrators anticipate demand and adjust staffing and supply orders accordingly.
Key results:
Better resource management
Fewer supply shortages
Improved staff morale with clear, data-driven planning
This example demonstrates how analytics can simplify planning and improve overall efficiency.
Results and Impact
Faster Insights, Better Decisions
AI-powered BI transforms data analysis, reducing the process from weeks to just minutes. This means healthcare providers can access insights quickly, leading to improved patient care and smoother operations.
Cost and Time Efficiency
In the three hospitals studied, AI-driven BI saved approximately $45,000 annually and significantly increased staff productivity by removing data bottlenecks. Below, we break down three steps to achieve similar outcomes.
Tips for Success
To achieve similar results, focus on these three steps, inspired by workflows from our hospital case studies.
Bring Your Data Together
Integrate systems like EHR, billing, radiology, and inventory into one platform. This allows AI-powered business intelligence to provide real-time, detailed insights. (Refer to Cases 1–3 for examples of improvements in billing, diagnostics, and staffing.)
Encourage Team Collaboration
Ensure clinical, administrative, and IT teams are aligned. Use AI tools that enable nontechnical staff to create their own queries and dashboards with ease.
Provide Targeted Training
Offer hands-on, role-specific training. Use conversational BI tools to help staff quickly access answers without relying on IT support. These steps lead to the efficiency, accuracy, and cost savings seen in the case studies.
Conclusion
AI-powered business intelligence has drastically reduced analysis time - from weeks to mere minutes - while improving both precision and independence. The challenge now is ensuring hospitals can maintain and build on these advancements.
Whether it's billing audits, diagnostic assistance, or staffing predictions, these examples showcase the transformative role of AI in healthcare. As the technology continues to advance, those who adopt it early are likely to set the pace for the future of patient care.
FAQs
What types of data can AI-powered business intelligence analyze in healthcare?
AI-powered business intelligence (BI) tools in healthcare can analyze a wide range of data to enhance decision-making and streamline operations. These tools typically process patient records, such as demographics, medical history, and treatment plans, as well as operational data like staffing schedules, resource allocation, and equipment usage. Additionally, they can handle financial data, including billing, insurance claims, and cost management.
By integrating and analyzing these diverse datasets, AI-driven BI platforms help healthcare organizations gain actionable insights, improve patient outcomes, and optimize operational efficiency.
What steps can healthcare organizations take to successfully implement AI-powered BI systems?
To ensure a successful implementation of AI-driven business intelligence (BI) systems, healthcare organizations should focus on a few key steps:
Define Clear Objectives: Identify specific goals, such as improving operational efficiency, enhancing patient care, or streamlining decision-making processes.
Ensure Data Readiness: Verify that data is accurate, well-organized, and compliant with healthcare regulations like HIPAA.
Engage Stakeholders: Involve both technical and non-technical staff early in the process to ensure smooth adoption and alignment with organizational needs.
Invest in Training: Provide comprehensive training for staff to effectively use the BI tools and interpret insights.
By following these steps, healthcare organizations can maximize the value of AI-powered BI platforms and drive meaningful, data-informed improvements across their operations.
What challenges might arise when using AI-powered business intelligence in healthcare?
While AI-powered business intelligence (BI) offers significant benefits in healthcare, there are potential challenges to consider. Data privacy and security are critical concerns, as healthcare organizations must comply with strict regulations like HIPAA to protect patient information. Additionally, implementing AI-driven BI platforms often requires high-quality, well-structured data, which can be difficult to achieve due to fragmented systems or inconsistent data collection practices.
Another challenge is the learning curve for staff. Adopting AI tools may require training and cultural shifts to ensure effective use and integration into existing workflows. Lastly, healthcare providers must address potential bias in AI models, which could lead to inaccurate insights or inequities in decision-making if not carefully monitored and managed.