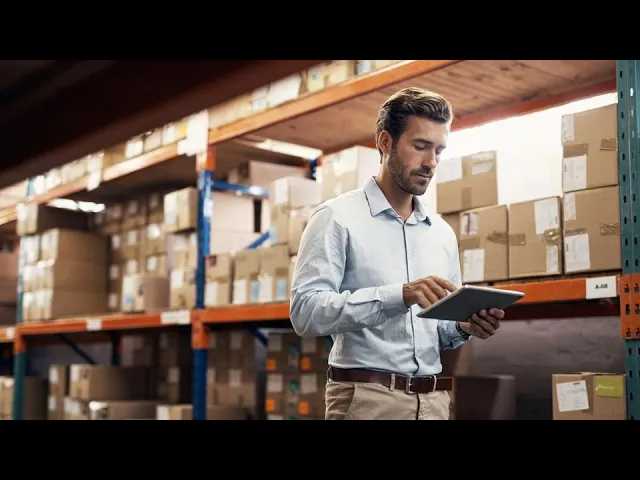
5 AI Use Cases for Supply Chain Data Integration
Supply Chain Management
May 20, 2025
Explore how AI enhances supply chain efficiency through improved demand forecasting, inventory management, risk analysis, logistics, and quality control.

AI is transforming supply chain management by solving data fragmentation issues and improving efficiency, accuracy, and cost savings. Companies adopting AI report reduced logistics costs (15%), lower inventory levels (35%), and improved service levels (65%). Here are the top five AI applications in supply chain data integration:
Demand Forecasting: Predict market needs with up to 50% higher accuracy, reducing stockouts and warehousing costs.
Inventory Management: Automate stock tracking and reordering, cutting carrying costs by 15–30%.
Supplier Risk Analysis: Detect and mitigate risks faster, saving up to 30% on disruptions.
Logistics Route Planning: Optimize routes to cut fuel costs, transit time, and emissions.
Quality Control: Achieve 99% defect detection accuracy, reducing waste and inspection time.
Quick Comparison
Use Case | Cost Savings | Accuracy Improvement | Efficiency Gains |
---|---|---|---|
Demand Forecasting | 20–50% inventory costs | Up to 98% forecast accuracy | 30% faster processing |
Inventory Management | 15–30% carrying costs | 85% stock accuracy | 40% reduced processing time |
Supplier Risk Analysis | 4% procurement savings | 90% risk detection | 50–70% faster assessment |
Logistics Route Planning | 15% transport costs | 95% route optimization | 22% reduced transit time |
Quality Control | 20% maintenance costs | 99% defect detection | 60% faster inspections |
AI is helping businesses like Amazon, Sanofi, and DHL achieve measurable operational and financial improvements, making supply chains smarter and more efficient. Ready to integrate AI into your supply chain? Start by assessing your current setup and identifying areas for improvement.
How AI is Revolutionizing Supply Chain Management (5 Minutes)
1. AI-Based Demand Forecasting
AI-driven demand forecasting has reshaped supply chain management, making it more efficient and responsive. According to McKinsey, businesses using AI forecasting tools have seen accuracy improve by 30-50% [3]. This goes beyond traditional methods, creating a supply chain that can adjust in real-time to market shifts.
Cost Savings
The financial benefits of AI forecasting are hard to ignore. Companies leveraging these systems have reported:
5-10% cuts in warehousing expenses
25-40% drops in administrative costs
Up to 65% fewer lost sales due to stock shortages [5]
These savings highlight how AI can streamline operations while reducing inefficiencies.
Prediction Accuracy
AI algorithms excel by analyzing complex datasets, including:
Historical sales trends
Macroeconomic data
Consumer sentiment
External disruptions [3]
Take Amazon as an example. Their AI systems maintain precise inventory levels while safeguarding profits with smart pricing strategies. By analyzing real-time and historical data - like customer purchase habits, seasonal trends, and regional demand variations - they fine-tune their forecasts to near perfection [3][4].
System Integration
The shift from traditional forecasting to AI-based methods is transformative. Here's a comparison:
Aspect | Traditional Forecasting | AI-Based Forecasting |
---|---|---|
Data Processing | Limited to structured data | Handles both structured and unstructured data |
Update Frequency | Weekly or monthly updates | Real-time, continuous updates |
External Factors | Basic consideration | In-depth analysis of weather, events, trends |
Accuracy Level | Moderate predictions | Up to 50% higher accuracy |
Time Reduction
AI forecasting tools also save time by automating up to 50% of repetitive tasks, leading to 10-15% cost reductions [5]. Siemens is a standout example: their machine learning algorithms analyze sensor data and maintenance logs to predict equipment failures before they happen [4].
Matt Wood, PwC’s US and Global Commercial Technology & Innovation Officer, underscores the rapid advancements in AI:
"AI adoption is progressing at a rapid clip, across PwC and in clients in every sector. 2025 will bring significant advancements in quality, accuracy, capability, and automation that will continue to compound on each other, accelerating toward a period of exponential growth." [5]
2. AI Inventory Management
AI-powered tools have completely reshaped inventory management by automating stock tracking and reordering processes. According to recent studies, 43% of small businesses either rely on manual methods to track inventory or skip tracking altogether [7]. This highlights a huge potential for AI to step in and revolutionize how inventory is managed, building on the demand forecasting advancements discussed earlier. The result? Streamlined operations across the entire supply chain.
Cost Savings
One of the biggest perks of AI in inventory management is its ability to save money. Here's how:
Cuts operational costs by 10–30% [8]
Reduces inventory holding costs by up to 25% [9]
Lowers storage expenses [6]
For example, a major manufacturer implemented an AI inventory assistant and saw a 15% reduction in excess stock, which directly slashed storage costs [6]. The system works by analyzing real-time data to keep inventory at just the right levels, all without human oversight.
Stock Accuracy
AI has also brought precision to inventory management, significantly improving stock accuracy:
Aspect | Impact |
---|---|
Forecast Accuracy | Up to 85% improvement [8] |
Stockout Reduction | Up to 65% decrease [8] |
Continuous IoT Tracking | Real-time tracking via sensors |
Automated Data Entry | Streamlined calculations and validation |
One global retailer rolled out an AI-powered inventory assistant across hundreds of stores and saw a 20% drop in stockouts, which led to happier customers [6].
System Integration
Modern AI inventory systems are designed to work seamlessly with ERP platforms, warehouse management systems, and IoT sensor networks. This integration creates a unified data flow, enabling real-time decision-making and full visibility across the supply chain.
Yatin Sapra, a Digital Transformation Consultant and Tech Blogger, puts it best:
"AI in inventory management is transforming how businesses handle stock. By automating tasks, predicting demand accurately, and reducing human errors, AI helps companies optimize stock levels, improve efficiency, and cut costs." [6]
Time Reduction
AI doesn't just save money - it saves time. Features like real-time monitoring, automated reordering, and predictive analytics drastically reduce the need for manual effort. These tools streamline procurement and boost efficiency throughout the supply chain.
Andy Moses, Senior Vice President of Solutions and Sales Strategy at Penske Logistics, sums it up:
"Machine learning and artificial intelligence will help you think faster and see your different options faster." [10]
With these capabilities, AI is redefining inventory management, making it faster, smarter, and more efficient for businesses everywhere.
3. AI Supplier Risk Analysis
AI has become a cornerstone in modern supply chain management. Inefficient procurement practices can drain up to 20% of budgets, making risk analysis a crucial tool to complement AI's role in forecasting, inventory management, and logistics improvements [12].
Cost Prevention
AI helps cut financial risks by automating the detection and mitigation of potential issues. For example, Everstream Analytics clients have reported notable savings:
Cost Area | Savings |
---|---|
Expedited Freight Costs | 5% reduction |
Revenue Loss from Disruptions | 30% reduction |
Temperature-Sensitive Freight | Over $2 million saved annually |
Disruption Assessment Time | 50-70% faster |
"Unlike humans, AI can process complex datasets efficiently, which can help with vendor selection and risk mitigation strategies" [13].
Improved Risk Detection
AI excels at identifying risks before they disrupt operations. It analyzes a wide range of data sources, including:
Financial reports
News articles
Social media sentiment
Regulatory databases
Weather patterns
Geopolitical events
"There's no need to manually comb through endless risk intelligence sources. NLP gets the job done much faster - surfacing insights they'd never catch on their own" [13].
Smarter Data Integration
Only 10% of companies have fully developed resilience capabilities [11]. AI-powered large language models simplify operations by allowing procurement teams to query contracts, suppliers, and spending data in plain language.
"This system incorporates a large language model that enables procurement professionals to ask questions about contracts, suppliers, and spending in plain language and receive instant answers. This setup lets their team focus on working with suppliers, driving innovation, and fulfilling stakeholder needs" [14].
By integrating supplier risk insights with other AI tools, businesses can create a more cohesive and proactive supply chain strategy.
Faster Response Time
AI doesn't just identify risks - it speeds up response times dramatically:
Traditional Approach | AI-Driven Approach |
---|---|
30-90 days for vendor assessments | Minutes to hours |
Manual delays | Real-time monitoring |
Weekly/monthly reports | Instant alerts |
Update frequency | 20-50x faster updates [15] |
"With predictive analytics, scenarios like this finally become preventable. This technology provides businesses with the foresight needed to stay agile and avoid being blindsided by unexpected supply chain disruptions" [13].
For instance, during a winter storm in Texas, a chemical company used AI to predict disruptions in their supply chain. The early warnings allowed them to act quickly, saving millions in potential losses [16].
4. AI Logistics Route Planning
AI-powered route optimization is changing the game for supply chain logistics by analyzing real-time data and complex variables. Businesses using AI routing solutions have seen logistics costs drop by an impressive 15% [18].
Fuel Cost Savings
One of the standout benefits of AI route planning is its ability to cut fuel consumption through smarter routing. Here's how it stacks up:
Optimization Factor | Cost Reduction |
---|---|
Annual Fuel Savings | 38 million liters |
Carbon Emissions | 1.5 million tons reduced |
Transit Time | 22% reduction |
Shipping Costs | 15% decrease |
Route Accuracy
DHL has taken delivery operations to the next level with its AI-powered forecasting platform, which operates in over 220 countries. By analyzing real-time traffic patterns, weather conditions, and scheduling data, the system achieves an impressive 95% prediction accuracy. This precision has also led to a 25% reduction in delivery times [17]. When combined with fleet integration, these accurate predictions significantly boost operational efficiency.
Fleet System Integration
Maersk provides a compelling example of how AI can revolutionize maritime logistics. Their advanced system processes over 2 billion data points daily from a fleet of more than 700 vessels. Here’s what they’ve achieved:
Predicts equipment failures up to three weeks in advance with 85% accuracy.
Reduces vessel downtime by 30%.
Saves over $300 million annually [17].
This level of integration ensures smoother operations and sets the stage for faster, more reliable deliveries.
Delivery Time
UPS’s ORION (On-Road Integrated Optimization and Navigation) platform is another shining example of AI in action [19]:
Performance Metric | Result |
---|---|
Route Optimizations | 30,000 per minute |
Driver Coverage | 35,000 of 55,000 drivers |
Cost Savings | $320 million |
Miles Saved | 10 million delivery miles |
ORION continuously adapts to real-time conditions, ensuring routes are as efficient as possible.
According to McKinsey, companies that have embraced AI in supply chain management early on have improved service levels by a remarkable 65% [20]. These advancements show just how much AI can enhance logistics, making supply chains faster, smarter, and more efficient.
5. AI Quality Control
AI is making waves in quality control, building on its success in forecasting, inventory management, risk analysis, and logistics. By leveraging advanced technologies, AI-powered systems are transforming how defects are detected - making the process faster and far more reliable. As of 2024, 63% of manufacturing companies have adopted AI for quality control processes [23].
Defect Cost Prevention
Catching defects early can save significant costs, and AI is proving to be a game-changer here. Take Ekoten, a leading textile manufacturer, as an example. By using Smartex's real-time AI inspection technology, they slashed defective fabric production by 80%. This system prevents over 4.5 tons of waste annually, addressing about 20 high-impact defects per machine each month [24].
Cost Reduction Metric | Industry Average Impact |
---|---|
Maintenance Costs | 20–40% savings |
Production Efficiency | 20–30% increase |
Safety Incidents | 40–60% reduction |
Downtime | 30–50% reduction |
Detection Accuracy
Manual inspections have long been the standard, but their defect detection rates typically range between 60% and 90% [21]. AI-driven systems, however, are raising the bar, achieving accuracy rates of up to 99% [25]. This heightened precision ensures that quality issues are addressed swiftly and effectively.
Production Line Integration
Major manufacturers are already seeing the benefits of integrating AI into their production lines:
Audi Neckarsulm Plant: By implementing AI for spot weld inspections, they cut labor costs by 30–50%, compared to traditional ultrasound testing [22].
Siemens: Their AI-powered computer vision systems detect subtle flaws - like dents, scratches, and misalignments - in real time [26].
Nestlé: AI sorting systems now identify contaminants and foreign objects in food products, boosting both safety and quality control efficiency [26].
These examples highlight how AI is speeding up inspection processes while improving accuracy.
Inspection Time
The impact of AI on inspection speed is striking. A leading car seat manufacturer adopted EasyODM's AI solution, reducing inspection time from 60 seconds per seat to just 2.2 seconds per seat. This shift also lowered defect rates by 30% and cut inspection costs by a staggering 30 times. The company achieved full ROI in under two years [21].
Results Overview
Integrating AI into supply chain operations brings measurable improvements across various areas. Let’s take a closer look at the key outcomes and their impact.
Benefits Breakdown
AI Use Case | Cost Reduction | Accuracy Improvement | Time/Efficiency Gains |
---|---|---|---|
Demand Forecasting | 20–50% inventory costs | Up to 98% forecast accuracy | 30% faster processing |
Inventory Management | 15–30% carrying costs | 85% stock accuracy | 40% reduced processing time |
Supplier Risk Analysis | 4% procurement savings | 90% risk detection | 50–70% faster assessment |
Logistics Route Planning | 15% transport costs | 95% route optimization | 22% reduced transit time |
Quality Control | 20% maintenance costs | 99% defect detection | 60% faster inspections |
The combined impact of these improvements delivers meaningful financial and operational gains.
Financial Impact Highlights
Take Amazon, for instance. Its AI-driven fulfillment centers improve productivity by 25–40% while reducing errors by as much as 90% [27].
"AI's ability to contextualize and interpret documents in real-time changes how we manage supply chain processes."
Ninaad Acharya, CEO & Founder, Fulfillment IQ [1]
Real-World Examples of Operational Success
Industry leaders showcase how AI transforms operations. Walmart, for example, uses AI to process 1.5 petabytes of data every hour across over 11,000 stores. This has led to a 16% reduction in out-of-stock situations, better inventory management, and improved customer satisfaction [27]. Similarly, companies like bp leverage AI for real-time tracking and optimization, demonstrating its value in enhancing operational performance [28].
Industry-Wide Impact
AI adoption is driving measurable improvements across the board:
Strategic sourcing: 3–8% procurement savings
Labor efficiency: 20–40% improvement
Profit margins: 7–10% higher than industry averages [27]
These results underline AI’s transformative role in reshaping supply chain processes for better efficiency and profitability.
Next Steps
If you're looking to integrate AI into your supply chain data systems, here’s a practical roadmap based on real-world examples of success.
Implementation Strategy
Assessment and Planning
Start by evaluating your logistics setup. Pinpoint areas that need improvement and establish baseline metrics to measure progress.
Technology Selection and Integration
Choose tools that align with your specific goals. For instance, DHL teamed up with Boston Consulting Group to refine their analytics processes, enabling quicker customer proposals. This kind of integration is essential for turning large data sets into actionable insights.
Data Management and Analytics
Tools like Querio can connect directly to your databases, simplifying complex supply chain data into insights you can act on through natural language queries.
Measuring Success
Once AI is up and running, keep track of its effectiveness by monitoring key performance indicators (KPIs). Here's how you can measure success:
Category | Key Metrics | Example/Target |
---|---|---|
Operational | Route Optimization | Over 10 million gallons of fuel saved annually [2] |
Financial | Cost Reduction | Around 15% cut in logistics costs [29] |
Efficiency | Processing Time | Noticeable time savings in real-world cases [29] |
Quality | Accuracy | Prediction accuracy improved from below 80% to over 90% [29] |
Real-World Success Stories
Real examples highlight how AI can transform supply chain operations. Take Mars Incorporated, for example. By working with Celonis, they used AI to analyze shipment details and even weather patterns to optimize truck loads. The results? An 80% reduction in manual work, lower shipping costs, and a smaller carbon footprint [2].
"The merger of human and machine is going to do great things for the business community. Machine learning and artificial intelligence will help you think faster and see your different options faster."
Andy Moses, SVP of Solutions and Sales Strategy at Penske Logistics [10]
Moving Forward
Continuous improvement is key. IBM, for instance, saved $160 million in supply chain costs by adopting cognitive supply chain technology [2]. This shows just how rewarding a well-planned AI strategy can be when it comes to long-term returns.
FAQs
How does AI enhance demand forecasting in supply chain management?
AI improves demand forecasting by diving into vast datasets, spotting patterns, and using real-time information to deliver sharper, more adaptable predictions. This means fewer forecasting mistakes, quicker responses to market shifts, and smarter decisions overall.
With tools like machine learning and predictive analytics, AI fine-tunes inventory management, cuts down on waste, and ensures products are stocked exactly where and when they're needed. It also adjusts to changes in consumer behavior and external influences, making forecasts more dependable and aligned with actual market conditions.
How can businesses save money by using AI in inventory management?
Integrating AI into inventory management can lead to significant savings, with businesses potentially saving $0.15 for every $1 spent. This happens by reducing surplus inventory and preventing stockouts. AI enhances demand forecasting, helping maintain the right stock levels, which lowers holding costs and improves cash flow.
Moreover, companies adopting AI have reported up to a 15% reduction in logistics costs and as much as a 35% improvement in inventory efficiency. These advantages make AI an effective way to optimize operations and increase profitability.
How can AI improve supplier risk analysis and help prevent supply chain disruptions?
AI takes supplier risk analysis to the next level by automating the review of massive datasets, including financial statements, market trends, and supplier performance indicators. This streamlines the process of spotting potential risks early, giving businesses the chance to address emerging challenges before they grow into larger problems. With continuous monitoring of supplier activities and external conditions, AI delivers real-time updates on shifting risk profiles, helping companies keep their supply chains steady and reliable.
On top of that, AI can identify patterns and connections between different risk factors, offering a clearer picture of supplier dependability. This added layer of insight allows businesses to make smarter decisions and take proactive steps to prevent disruptions. The result? Smoother operations and stronger, more reliable partnerships throughout the supply chain.